Hongseok Namkoong
Assistant Professor, Columbia University
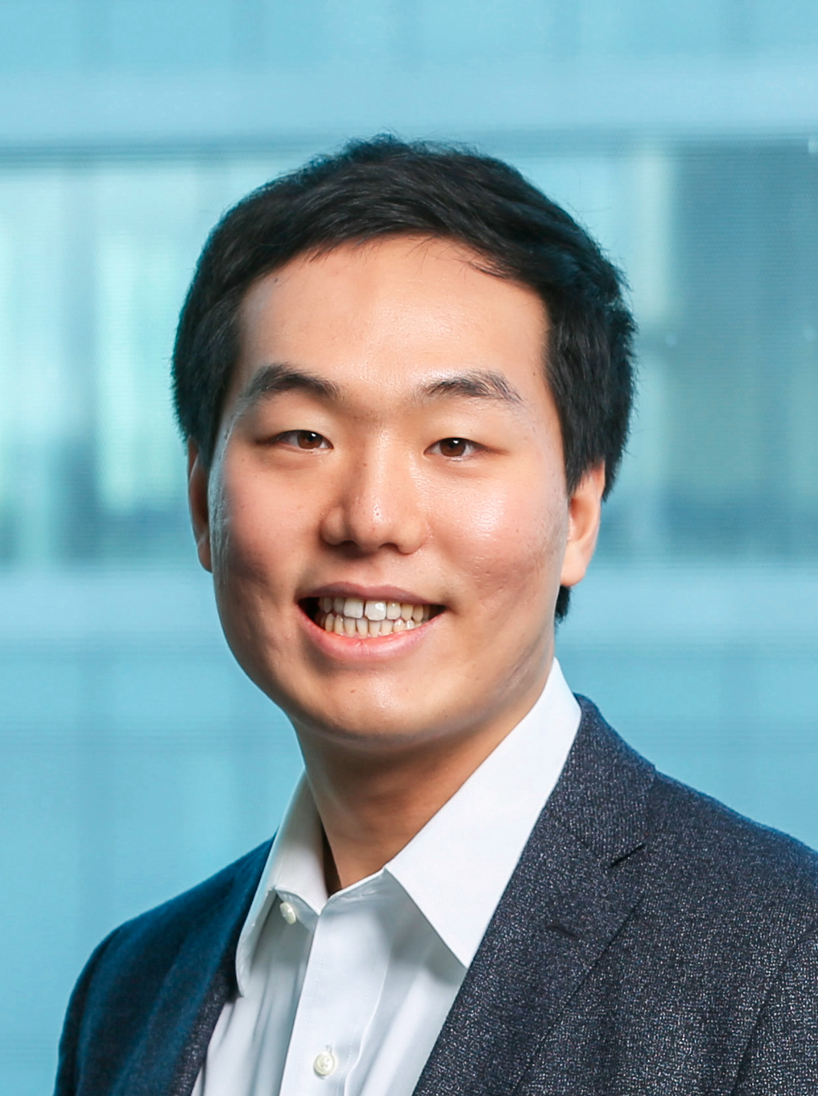
namkoong@gsb.columbia.edu
I am an assistant professor in the Decision, Risk, and Operations division at Columbia Business School and a member of the Data Science Institute. I work on building trustworthy AI systems that are capable of solving real-world decision-making problems. I take a data-centric view of AI systems, and am a strong believer in algorithmic ideas simultaneously grounded in empirical foundations and principled thinking. As an interdisciplinary researcher, I connect and extend tools from machine learning, operations research, and statistics. Read this overview of my research to learn more about my impact-driven agenda.
Before joining Columbia, I received my Ph.D. from Stanford University and spent a year at Meta’s Adaptive Experimentation team as a research scientist. Outside of academia, I serve as a LinkedIn Scholar at LinkedIn’s Core AI team. Here’s a more formal bio in the third person. I go by Hong; alternatively, here’s a link the correct pronunciation of my first name.
news
Feb 14, 2025 | I’m looking for motivated undergraduate and master’s students to work on ML research. Fill this form out if you’re interested. |
---|---|
Dec 05, 2024 | AI systems are omni-present, yet extrapolate unreliably. Improving AI safety and capabilities hinges on comprehension of uncertainty and actively making decisions to resolve it. Instead of cumbersome probabilistic models, my team leverages a predictive view of uncertainty to build a scalable framework based on autoregressive models. Watch this recent Simons talk to learn more. |
selected publications
-
Trustworthy AIarXiv:2503.21023 [cs.LG], 2025
-
AI-driven DecisionsarXiv:2405.19466 [cs.LG], 2025Selected for presentation at the Econometric Society Interdisciplinary Frontiers: Economics and AI+ML conference